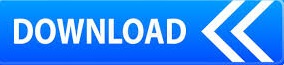
_thumbnail.jpg)
Demographic features and location features are also extracted. In this paper, we propose a personalized location recommendation method using mobile phone usage information, which transforms the location recommendation problem into a regression task, and extracts six types of mobile phone usage features to profile users. In addition, abundant mobile phone usage information can be recorded when users are using their phones, e.g., the use frequency of Apps, which can fully reveal the diverse characteristics of different users. However, such little information is not sufficient to profile users accurately. To address this problem, the existing studies usually exploit other information, e.g., demographic features, to characterize users. The cold-start problem is still a great challenge in personalized location recommendation, which makes it difficult to infer a new user’s preferences, because a new user generally has never visited any location at the start. Location recommendation has become a hot research area in recent years. The results show that CoSafe achieves a mean false negative ratio and false positive ratio of 1.46 and 3.12 percent, respectively, even under sophisticated stealing attacks.
IGUARD 210 DIRECT IMAGE ANDROID
We implement CoSafe on a Commercial-Off-The-Shelf Android smartphone and a smartwatch, and conduct both trace-driven simulations and real-world experiments to evaluate the performance of CoSafe. In this way, CoSafe can instantly respond and introduce very low computation and communication costs.
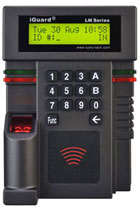

Specifically, CoSafe compares the mobility consistency between a pair of devices on three levels, where coarse features (i.e., the mobility state and motion periodicity) are first compared to give fast response and more complex comparison on subtle feature (i.e., the relative phase) is conducted only when needed. The rationale is that the vibrations perceived on devices carried by the same person should be tightly coupled whereas a lost device would show distinct mobility characteristics from others. , to detect device loss by verifying the motion consistency between a pair of devices. Noticing the trend that one person normally carries more than one mobile device, we propose an innovative scheme, called As mobile devices play increasingly important roles in our daily lives, it is of great significance to protect personal mobile devices from being lost.
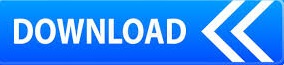